Fearfully intelligent? It’s not quite as scary as Hollywood and science fiction describe AI technologies after all. Artificial intelligence (AI) merely automates learning through cleverly selected repetition operators. The goal here is to use AI to perform computer-controlled tasks reliably and without fatigue. Another point that shows AI is not scary is that we still need human intelligence for setting up and determining the right question. AI systems can only be trained to perform a clear task. For example, a poker AI can never play chess or solitaire.
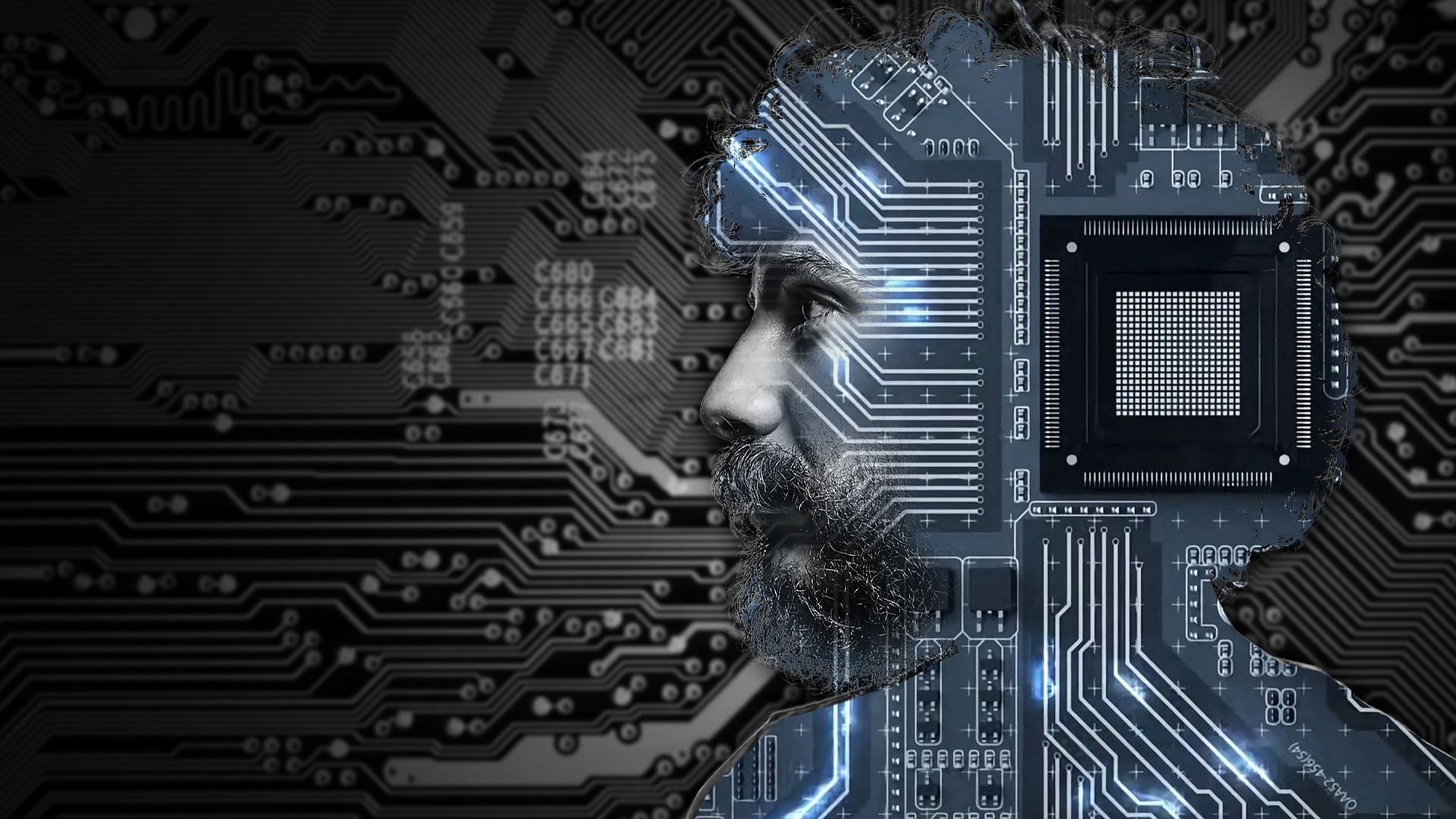
AI can only be implemented with the help of human intelligence so far
How important is data in relation to AI?
However, these issues are not barriers to the effective application of AI. In medicine, AI methods from the fields of deep learning, machine vision and object recognition already achieve the same accuracy in diagnosing cancer in MRI images as a well-trained radiologist. AI algorithms can even predict waves of disease: for example, a Canadian company’s algorithm warned nine days before the WTO that an epidemic was about to break out in Wuhan. Chatbots are also an effective AI technique. These programs simulate a conversation with a human online. Self-driving cars also represent an AI application.
Here, the amount of data plays a bigger role than ever before. Error-free data quality is crucial to turning Big Data into structured Smart Data. If the values are not normalized, incorrect or outdated, this severely limits the performance of the system. In the worst case, this can lead to incorrect conclusions. For example, incorrect data can leave a patient’s cancer undetected.
In industries with high competition, the companies with the best data win – even if their competitors use similar methods. This is because AI learns based on the data. Its results directly reflect its accuracy.
Areas of application for AI
A serious challenge is the disparity in assessments of ML within their own company between management, IT departments and business units. Over half of business leaders believe that a number of AI applications are already in use in their companies. However, only just under 20% of IT professionals share this opinion. Sufficient exchange of information? Missing.
But which systems are presenting companies with these communication problems? The top four AI applications in use:
- Machine learning and reasoning (55%),
- Machine vision (49%),
- knowledge-based systems (46%),
- Speech and text understanding (30%).
Large enterprises mostly focus on face recognition, optical drawing, and planning systems when using AI systems. Medium-sized companies use AI almost exclusively for behavior-based prediction and audience identification. For small companies, the main focus in ML is on Big Data & Analytics and Hyper Targeting. Thus, it can be seen that small and medium-sized companies use AI primarily to optimize customer orientation and customer service.
These applications are usually not possible without external help, as there is often a lack of know-how and corresponding skilled personnel. Small companies are more willing to buy in knowledge for special ML applications. 39% of small companies work with around four to five external service providers. Large and medium-sized companies limit themselves in most cases to two to three external service providers.
In 2019, price was the most important selection criterion for AI technologies from external service providers. This has changed in 2020, as decision-makers place higher value on ease of use and a comprehensible way of working. The trend reflects the negative experience to date.
How is Germany developing in terms of AI?
In a recent study by PwC, 70% of respondents see information technology professionals as responsible for ensuring that data has no errors. 57% see management as responsible for preventing them. 22% of respondents assign this task to software suppliers and 20% name data analysts. In the opinion of the respondents, 72% want specialist managers in the IT area to prevent damage that arises in connection with AI. Here, management bears a significantly smaller responsibility (33%) and data analysts a slightly higher responsibility (27%).
In 2019, ICT companies spent a full 0.56% of their revenue on the development, introduction and maintenance of AI processes. Business-related services (0.29%) and mechanical/electrical engineering (0.17%) also provided relatively high shares of their total sales. In comparison, the overall economic share is only 0.09%.
Even before 2010, one in five of the AI-using companies used artificial intelligence for the first time. Another 20% used AI for the first time in the first half of the 2010s. After 2015, the use of AI accelerated significantly. One-third of companies using AI in 2019 first adopted it between 2016 and 2017. The remaining 27% of AI-using companies joined in 2018 and 2019.
German companies have made year-over-year advancements in machine learning (ML) and artificial intelligence. According to a study by IDG, three-quarters of the companies surveyed are already using AI or are in the process of introducing it in 2020. This is 20% more companies than in the previous year. A large proportion (70%) are noticing positive effects of AI no later than six months after its introduction.
What potential is there in AI?
And yet it moves. That describes the current movement of companies toward artificial intelligence and machine learning well. However, a little more movement wouldn’t hurt many companies, as they are very hesitant to enter the world of AI.
Doing without Artificial Intelligence, Machine Learning and Deep Learning is not an option. The abundance of applications from German companies and research institutes, such as the Frauenhofer, Max Planck or Helmholz communities, makes this clear. Typical examples are the automatic inspection of ball screw gears in machine tools or the defense against cyber attacks. Thus, AI, ML and Deep Learning have become indispensable to modern concepts.
The Silicon Valley giants may have big power and big money, but in this country, the majority of mechanical engineers are much better educated. Even today, over 50% of the world’s patents in the field of autonomous driving are still attributable to companies based in the Munich area. The Bundeswehr University in Neubiberg plays a crucial role here.
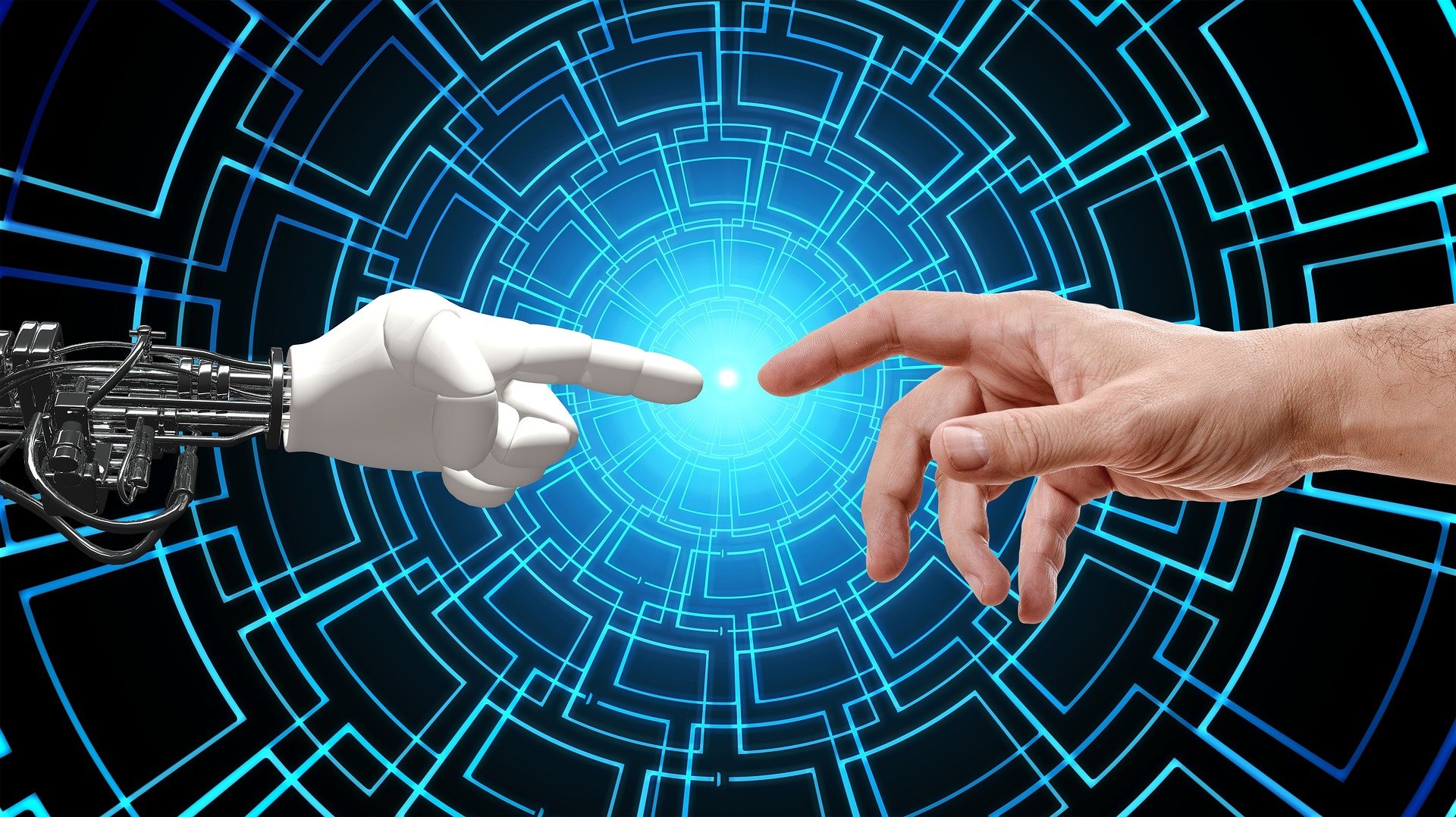
Bringing people and technology closer together
For companies, therefore, there is no way around an intensive examination of these technologies. Friction losses due to exaggerated expectations, hesitant approaches and communication problems should therefore be stopped as soon as possible. Otherwise, “And yet it doesn’t move” would soon become an unwelcome reality.
So how can companies positively influence their urge to move?
The path to success
Companies strive for quick success. However, they often don’t yet consider ML technologies as a way to use them to expand their product and service portfolios. One such component is the automatic storage of washing habits. Here, frequently used programs are automatically suggested by the washing machine for each wash cycle. Companies without foresight are thus unnecessarily missing out on many opportunities for themselves and their customers.
Germany is on a par with other countries when it comes to implementing artificial intelligence. Nevertheless, there is still a need for optimization in some areas of action:
- Implement overarching strategies: The time for pilot projects is over. Companies should move on to designing overarching AI strategies and implementing them company-wide.
- Make targeted use of external expertise: Germany already relies on external AI partners, resulting in faster implementation and lower costs. Given the shortage of skilled workers, this is feasible and makes sense even for smaller companies.
- Embrace competition and skilled workers: Skills such as data science, AI research and AI project management are currently in particularly high demand and will be essential in the long term. If a company wants to survive this competition, internal AI skills are needed.
- Improve risk management: German companies should improve internal structures, skills and processes to minimize AI risks and position themselves more broadly with the installation of guidelines, control regimes and risk officers.
AI systems are trained to do one thing at a time. As the computing power of computers continues to grow, in as little as three decades, computers the size of smartphones will have reached the computing power of the human brain. This means that AI applications will run ever more smoothly and quickly. But even then, human intelligence still remains necessary to set up AI, Deep Learning and Machine Learning.
(Source: Deloitte)